The AI workforce is a team of diverse AI agents equipped with AI tools. These agents work collaboratively in a multi-agent system to solve complex tasks.
The pace of innovation in artificial intelligence is accelerating at an astounding rate. Technologies that were once only theoretical are now becoming practical realities. Companies across industries are increasingly adopting AI to automate tasks, enhance productivity and create new possibilities.
There is one particular area in AI that has even captured the fascination of legendary technologists like Bill Gates and Vinod Khosla, that is AI agents - A type of AI system that is designed to act autonomously and accomplish even complex tasks on behalf of a user, it is able to plan, reason, react and make actions.
In less than ten years each American will have a dozen or more AI agents constantly running around the net with AI that is 10x more powerful.
- Vinod Khosla, early investor in OpenAI, founder of Suns Microsystems and Khosla Venture.
Agents are coming. In the next few years, they will utterly change how we live our lives, online and off.
- Bill Gates, Founder of Microsoft.
With this increasing adoption and development of AI agents, the birth of a new segment to the workforce is becoming increasingly important - the AI workforce. Every company will have not just a human workforce but also an AI workforce working in tandem. Teams will only be limited by their ideas, not their size.
How do you build an AI workforce?

To build an AI Workforce there are three core building blocks to it:
- AI agents: they are digital workers that given instructions will complete a task. These AI agents are powered by LLMs such as GPT, Mistral, Claude and more. These need to be meticulously created to be effective. They need to be exceptionally well-instructed and well-constructed so they can run with any technical errors. This is before we get towards human level reasoning, thoughts, and actions
- Tools for the Agents: In order for Agents to ascend from just generating text it needs to be given access to AI tools such as “google search”, “use salesforce”, “run code” to give it real superpowers to automate tasks.
- Multi-agent system: In order for agents to work collaboratively like a team, you’ll need a multi-agent system, which allows different types of diverse agents to interact and speak with each other. A team of AI agents will always be more effective than 1.
An effective AI workforce is one that has a diverse team of AI agents that are domain experts, each equipped with powerful and relevant tools for that domain and working in a multi-agent system.
What are AI Agents?
AI agents can be broadly classified into two categories based on their level of autonomy and the nature of their interaction with users: Co-pilot and Autopilot.
Co-pilot Agents - AI in the Loop
Co-pilot agents are a widespread form of AI agents, designed to work alongside humans and augment our productivity. AI in the loop refers to the practice of actively involving AI systems in the decision-making process, while still maintaining human oversight and control. They are integrated into various tools and platforms, assisting with tasks and streamlining workflows. In fact, according to a recent report, the global AI co-pilot market is projected to reach $23.1 billion by 2027, growing at a Compound Annual Growth Rate (CAGR) of 38.4% during the forecast period. Additionally, a survey by Gartner found that 75% of organizations have already deployed or plan to deploy co-pilot agents in the next year.
Here are some examples of co-pilot agents in use:
- Github Co-pilot for coding. Embedded directly into visual studio code and github to help developers write effective code more quickly and focus on the core parts of building durable software such as logic.
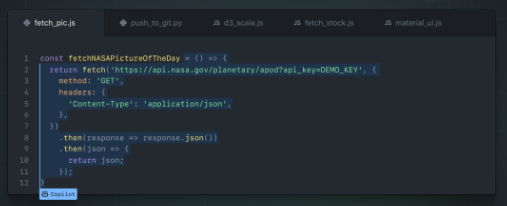
- Microsoft 365 Co-pilot for presentations. Embedded directly into Microsoft suite like Microsoft PowerPoint to help you create PowerPoints more effectively

- Notion AI for writing. Embedded directly into notion to help you create notion documents more effectively.
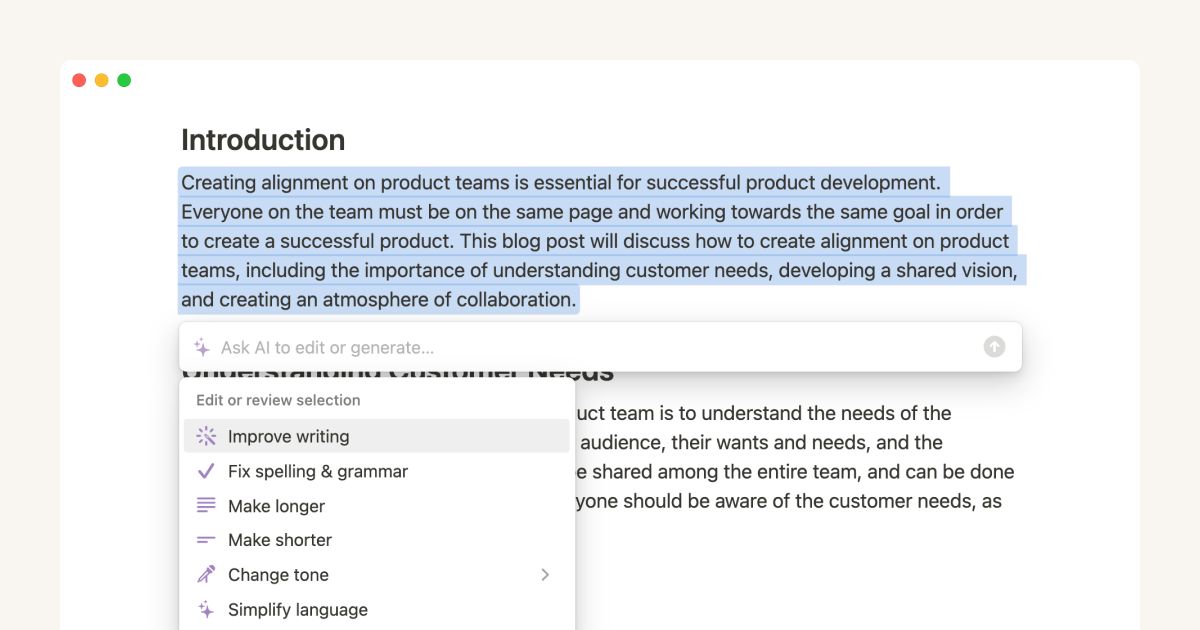
Autopilot Agents - Human in the Loop
Autopilot agents different to co-pilots in that they are designed to act autonomously, interacting with tools as a human would, and instead require humans to supervise or manage them. Autopilot agents represent a significant advancement in AI technology, capable of autonomously completing tasks with minimal human intervention. However, even with their advanced capabilities, these agents are not yet perfect and sometimes require human oversight to ensure accuracy and precision. This is where the "human-in-the-loop" approach comes in, allowing humans to review and correct the decisions made by autopilot agents. This collaborative approach enables the benefits of automation while still leveraging human judgment and expertise, and is particularly useful in high-stakes applications such as healthcare, finance, and transportation.

Klarna recently announced that their customer support autopilot chatbot handled 2.3 million customer service chats in 35 languages worldwide during its first four weeks. This volume equates to the workload of 700 full-time human agents. Klarna reported that their autopilot achieved customer satisfaction ratings equal to its human team members. It also showed higher accuracy than humans with a 25 per cent reduction in repeat inquiries. Moreover, it can resolve tickets in less than 2 minutes, compared to the previous benchmark of 11 minutes.
AI in the Loop (AITL) versus Human in the Loop (HITL)
As AI technology continues to advance and become increasingly integrated into various aspects of our lives, it's essential to understand the different approaches to human-AI collaboration. Two prominent paradigms have emerged: Human in the Loop (HITL) and AI in the Loop (AITL). While both approaches aim to leverage the strengths of humans and AI, they differ significantly in their underlying philosophy, application, and benefits.
Key differences:
- Level of autonomy: HITL has higher autonomy, as AI makes decisions and humans only review and correct when necessary, whereas AITL has lower autonomy, as AI acts as a co-pilot, providing recommendations for human decision-making.
- Role of humans: In HITL, humans have a reviewing and correcting role, while in AITL, humans have a decision-making role, with AI providing support.
- Decision-making process: HITL involves AI making decisions, followed by human review and correction, while AITL involves humans making decisions, with AI providing recommendations.
- Applications: HITL is suited for applications where AI is highly advanced and accurate, such as data processing and analysis, while AITL is suitable for complex decision-making and creative tasks.
- Benefits: HITL ensures efficiency and accuracy, while AITL enhances human capabilities and provides additional insights.
How to make an effective agent?
Creating an effective AI agent requires a thoughtful and systematic approach. It involves combining cutting-edge technologies like machine learning and natural language processing with a deep understanding of the agent's intended goals, tasks, and environment. By carefully designing and training an AI agent, developers can unlock its full potential and create a powerful tool that enhances productivity, decision-making, and overall performance.
.png)
There are 5 core components:
Large Language Model (LLM)
At the heart of any AI agent is a powerful foundational model, usually what's known as a large language model. Unlike simple rules-based chatbots, LLMs like GPT4 and Anthropic's Claude can understand nuanced language, generate coherent long-form text, and even execute logical reasoning when prompted appropriately.
System Prompt
The system prompt serves as the personality and reasoning patterns for the AI agent. Carefully engineered prompts prime the LLM to respond appropriately as that agent, whether as a helpful assistant, curious child, or domain expert. There are many kinds of different system prompts that can enable different kinds of reasoning behaviour such as Chain of thought, Tree of thought, ReAct and more.
Memory
In addition to analyzing the current input text, effective AI agents need memory of past interactions and context. This could be a simple short-term memory to recall the last few exchanges or a more comprehensive long-term memory component. With memory, agents can have true conversations that reference shared history and maintain logical consistency.
Feedback
It is crucial that the Agent is receiving feedback from the user on good and bad actions. This feedback can then be incorporated via fine-tuning the model using methods like RLHF.
Tools for agents
While LLMs can generate remarkable text, agents need additional tools to take meaningful actions. This could include APIs to look up information, integrate with calendars, or complete transactions. With tools, agents can go beyond chat to truly assist users across applications.
Together, these four components enable AI agents that understand, learn, reason, and act in an intelligent manner. The rapid evolution of these core capabilities is bringing us closer to artificial general intelligence. The next generation of AI agents will collaborate with humans seamlessly
Tools for AI agents - Giving Agents the ability to interact with the world
In order for agents to ascend from just generating text and interacting with the real world, it needs to be given access to tools.
For those with an engineering background you can think of tools like functions. They are ‘skills’ that an agent can call and execute when it deems the task requires it.
There are 3 core types of tools that is useful in making an agent powerful.
- Source of truth tools. These are knowledge bases, files where the agent can access data about different topics to answer new questions. E.g. “Legislation lookup”, “HR manual”

- Functional tools. These are APIs / integrations to run some sort of action. E.g. “Send an email”

- Skill based tools. These are use case specific workflows constructed by domain experts that can be made up of multiple LLMs each with expert crafted prompts, and other tools. E.g. “How to reply to an NDA request professionally.”

Here are some examples of tools being utilized in both a co-pilot and autopilot system:
This co-pilot example shows a tool that can find the job title, company, and company size of a person given an email address. This is a GPT built in ChatGPT using our Custom Actions for GPTs feature.
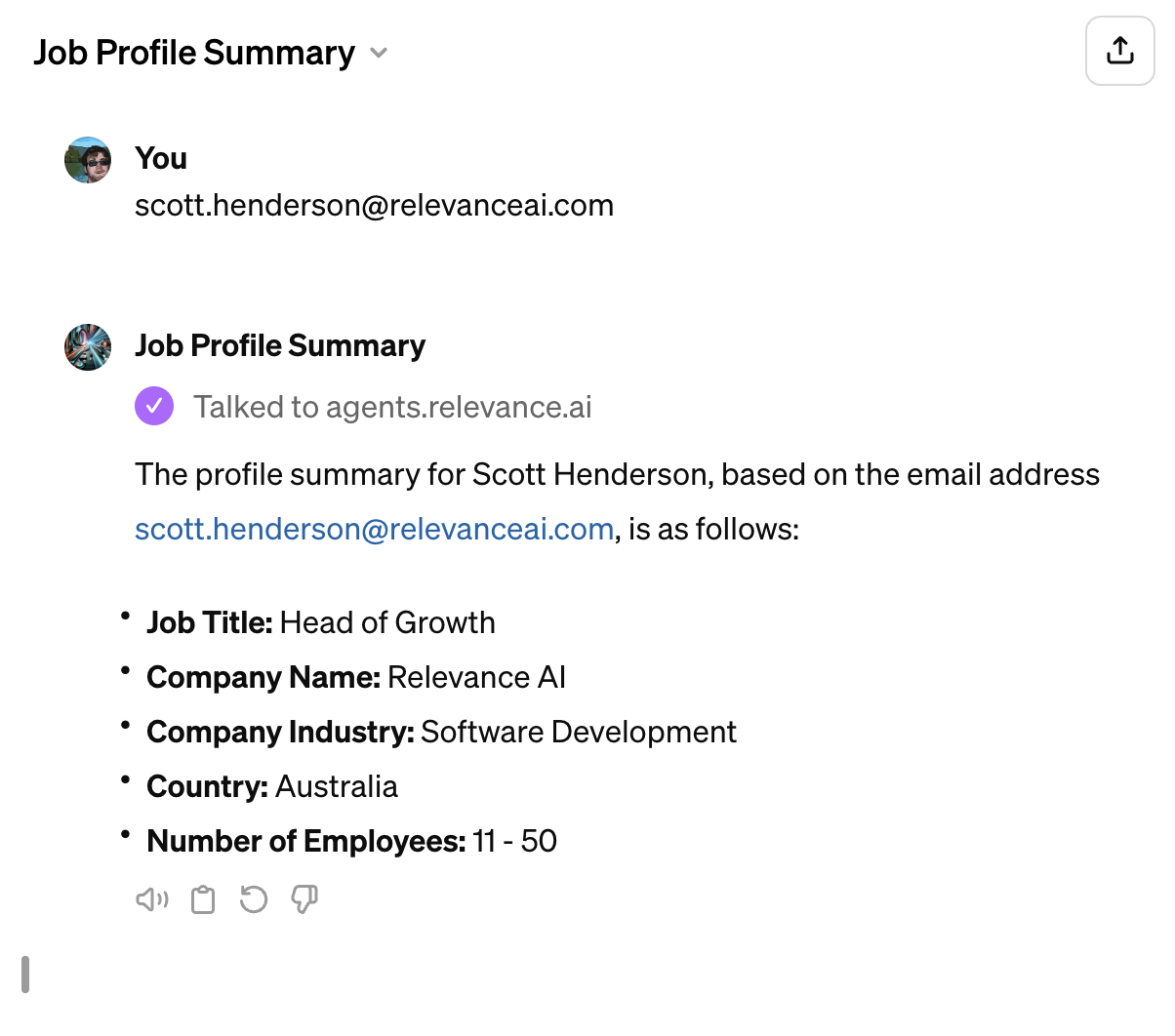
This auto-pilot example shows a writing agent that has the tools for Google Search and Website Scraping. So they can do desk research using Google and then scrape the content from the results to summarize and help them write an article. This is built in Relevance AI.
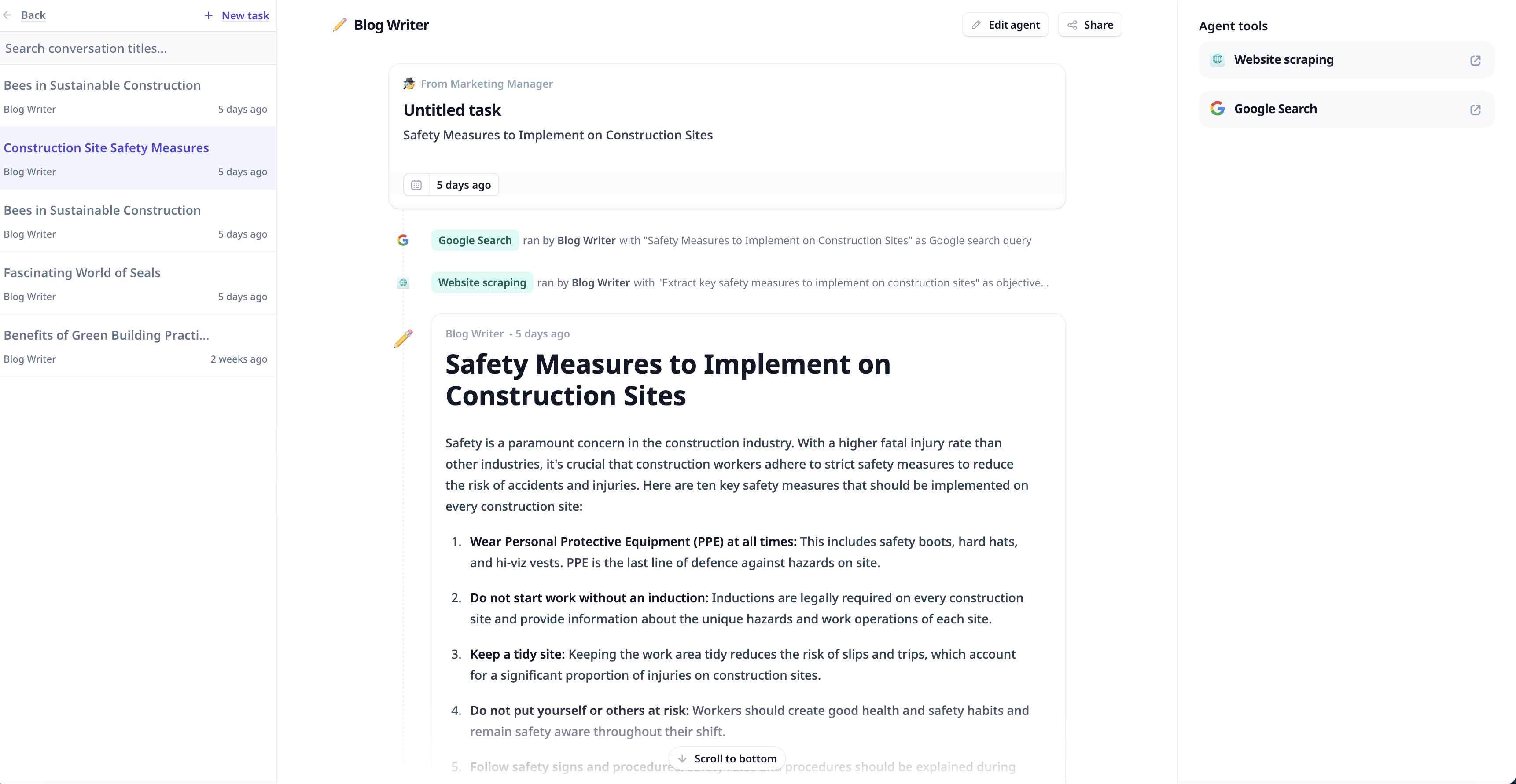
What are Multi-agent Systems?
Often the work we do in teams require expertise from different disciplines in order to effectively complete a task. For example when you are launching a very simple feature of a product you need. A product marketer to manage the launch, a content creator to write a landing page and help documentation, a designer to design the landing page, a data analyst to report on it’s usage so you can see if it’s been effective. If you only had the skills of a content writer on this project, you would miss out on the benefits of visual communication and the feedback loop you get from data.
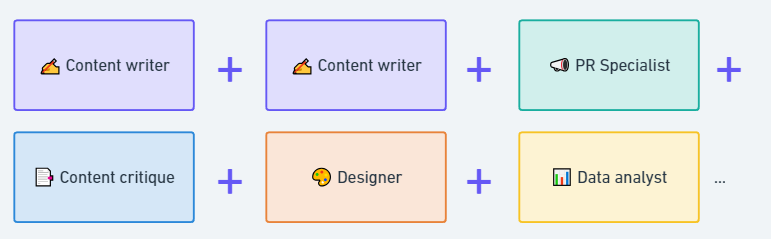
❌ Avoid using any agents in that can only operate in silos you would be missing majority of the compounding effects that the AI workforce can bring.

In order for agents to be effectively work on complex tasks, you need a multi-agent system. There are many different types of multi-agent system. Here are some examples:
Assembly line - Each agent completes their task sequentially given a SOP. Great for process driven task where the process is well figured out but requires a lot of reasoning to decide which path of a process to go down.

Omni-direct - All agents are in 1 meeting room constantly speaking to one another. Great for creative tasks.

Leader driven - A leader agent creates and adjusts the plan and coordinates majority of the communication and subtasks to be completed. Its best when both creativity and process is required.

AI and the Workforce
The integration of Artificial Intelligence (AI) into the workforce presents both challenges and opportunities. On one hand, AI has the potential to automate routine and repetitive tasks, freeing up human workers to focus on more complex and creative tasks. On the other hand, there is a risk that AI could displace certain jobs, particularly those that involve tasks that are easily automatable. According to a report by Gartner, by 2025, 14% of the global workforce will be either augmented or replaced by AI.
Despite these challenges, significant progress has been made in recent years to prepare the workforce for an AI-driven future. Many organizations are investing in upskilling and reskilling programs to help workers develop the skills they need to work effectively with AI. Additionally, governments and educational institutions are launching initiatives to promote STEM education and digital literacy. Looking ahead, the future of work with AI holds much promise. As AI takes over routine tasks, workers will be able to focus on higher-value tasks that require creativity, empathy, and problem-solving skills. According to a report by the World Economic Forum, by 2022, 75 million jobs may be displaced, but 133 million new roles may emerge that are more adapted to the new division of labor between humans, machines, and algorithms.
The Growing Impact of AI on the Modern Workforce
Recent studies indicate that AI is reshaping the global workforce at an unprecedented pace. As of 2024, approximately 77% of businesses are either actively integrating AI or exploring its implementation, with larger enterprises showing twice the adoption rate of smaller businesses. This transformation is creating both challenges and opportunities across industries.
Key statistics highlight that:
- 30% of workers globally anticipate AI impacting their roles within the next three years
- AI is projected to influence approximately 800 million jobs worldwide by 2030
- 81% of office workers report improved job performance through AI integration
- Organizations are investing heavily in AI workforce development, with major companies like Accenture pledging to double their AI workforce to 80,000 professionals
The Evolution of AI Workforce Systems
The AI workforce landscape continues to evolve rapidly, with several emerging trends shaping its future:
Emerging Trends:
- Hybrid Workforce Models: Integration of human workers with AI systems in complementary roles
- Personalized AI Assistants: Development of AI agents tailored to specific job functions and industries
- Enhanced Collaboration Systems: Advanced multi-agent systems enabling more complex task completion
- Adaptive Learning Capabilities: AI systems that continuously improve through interaction and feedback
Industry-Specific Applications:
Different sectors are developing specialized AI workforce solutions:
- Healthcare: AI agents for patient care coordination and medical data analysis
- Financial Services: Automated risk assessment and customer service systems
- Manufacturing: Smart production systems and quality control
- Professional Services: AI-enhanced research and analysis tools
Preparing for the AI-Enhanced Workplace
The transition to an AI workforce requires a comprehensive approach to skills development and training. Research shows that over 120 million workers globally will need retraining in the next three years to effectively work alongside AI systems.
Essential skills for the AI-enhanced workplace include:
- Technical Competencies
- Understanding AI fundamentals and capabilities
- Data literacy and analysis
- Digital tool proficiency
- AI system monitoring and maintenance
Human-Centric Skills
- Critical thinking and problem-solving
- Emotional intelligence and interpersonal communication
- Ethical decision-making
- Adaptability and continuous learning
Organizations must invest in comprehensive training programs that balance technical knowledge with human-centric skills to create a workforce that can effectively collaborate with AI systems.
Navigating AI Workforce Implementation
While implementing an AI workforce offers significant benefits, organizations must address several key challenges:
Common Implementation Challenges
• Skills gaps and training requirements• Integration with existing systems and processes • Data privacy and security concerns • Employee adoption and change management • Ethical considerations and bias mitigation
Successful Implementation Strategies
- Phased Approach: Begin with pilot programs in specific departments before expanding
- Comprehensive Training: Develop structured training programs for all employee levels
- Clear Communication: Maintain transparent communication about AI implementation goals and impact
- Regular Assessment: Monitor and evaluate AI system performance and workforce adaptation
- Ethical Framework: Establish clear guidelines for AI use and decision-making
Responsible AI Workforce Development
Organizations must prioritize ethical considerations in AI workforce implementation:
Key Ethical Considerations
- Transparency in AI decision-making
- Fair and unbiased system operation
- Privacy protection and data security
- Human oversight and control
- Responsible automation practices
Governance Framework
- Clear policies for AI use and deployment
- Regular ethical audits and assessments
- Stakeholder engagement protocols
- Compliance with regulatory requirements
- Employee rights and protections
These comprehensive additions provide a well-rounded view of the AI workforce landscape, incorporating current research, practical implementation guidance, and future-focused insights. The content maintains professional depth while remaining accessible to readers of varying technical backgrounds.
Creating an AI workforce
The importance of an AI workforce and AI agents in today's world is growing rapidly. As artificial intelligence continues to advance, companies are harnessing the power of AI agents to automate tasks, increase productivity, and even create new possibilities. These AI agents, acting as digital workers, are capable of performing complex tasks autonomously, contributing to the emerging concept of an AI workforce. Such a workforce, which works in parallel with human teams, is becoming an essential component in many industries, revolutionizing the way we work and conduct business.
Creating an effective AI workforce involves numerous critical elements to ensure the efficiency of each AI agent. This necessitates the human workforce to encapsulate their domain expertise into prompts and tools, while continuously observing and providing feedback. Doing so allows the human workforce to delegate highly repetitive tasks to the AI agents, enabling them to focus on tasks that only humans can perform.
However creating an AI workforce can be easier, thats why we have built at Relevance AI. You can sign up here and try it out.