From Tools to Teams: Unlocking Relevance AI’s Full Potential
This is a guest post from Henry LeGuard, CEO of Verisoul. Verisoul is an AI platform that helps businesses automate prevention of fake accounts like duplicates, bots, spammers, and fraudsters.
At Verisoul, we prioritize “return on time” when evaluating any new GTM initiative. We’re a 2-person growth team, primarily constrained by the # of hours in a day. The seemingly boundless number of GTM opportunities leads to trade-offs: should we customize every email, or focus on quantity over quality? Should we post relevant content on LinkedIn weekly, or draft thought pieces for newsletters monthly? As a team itching to show off our product to the world, we were ecstatic to add a new productivity hack to the team: Relevance AI. In a matter of weeks, our team of 2 started operating like a team of 20.
Today, we’ll discuss how our growth team quickly evolved from the most basic building blocks of Relevance AI - the tools - to building teams of agents triggered by automated workflows.
Verisoul’s early Relevance AI use cases
Within days of setting up Relevance AI, the power of the workflows was apparent. With just three tool steps - google search, website scrape, and ChatGPT - we were able to create a variety of tools that enabled our daily outreach campaigns:
- List scraping tools to identify sets of companies
- LinkedIn URL finders, allowing us to drop in the above set of companies and identify the right person to reach out to at each company
- Personalized messages to prospects via LinkedIn scraping, company website scraping, and Relevance AI’s knowledge-base of Verisoul’s offering
Among many others. While we eventually augmented our product with some of the other 100-odd tool steps, these three allowed us to hit the ground sprinting. The outcome when combined with 6Sense and Amplemarket? Hundreds of custom emails sent to individuals interested in purchasing fake account prevention software weekly.
Side note: Relevance AI vs. Copy AI
We tried using competitors like Copy AI, but struggled with the user interface and the overall guardrails and training wheels on the platform. Even creating a single tool meant relying on an LLM to appropriately interpret our commands and produce the proper workflow prompts. Relevance AI, on the other hand, had intuitive piping structures, far more capabilities, and a more open framework, which allowed us to directly make the updates ourselves. Copy AI’s inability to search Google might’ve been the largest limitation, a feature used in >50% of our Relevance AI tools.
From Tools, to Agents, to Teams
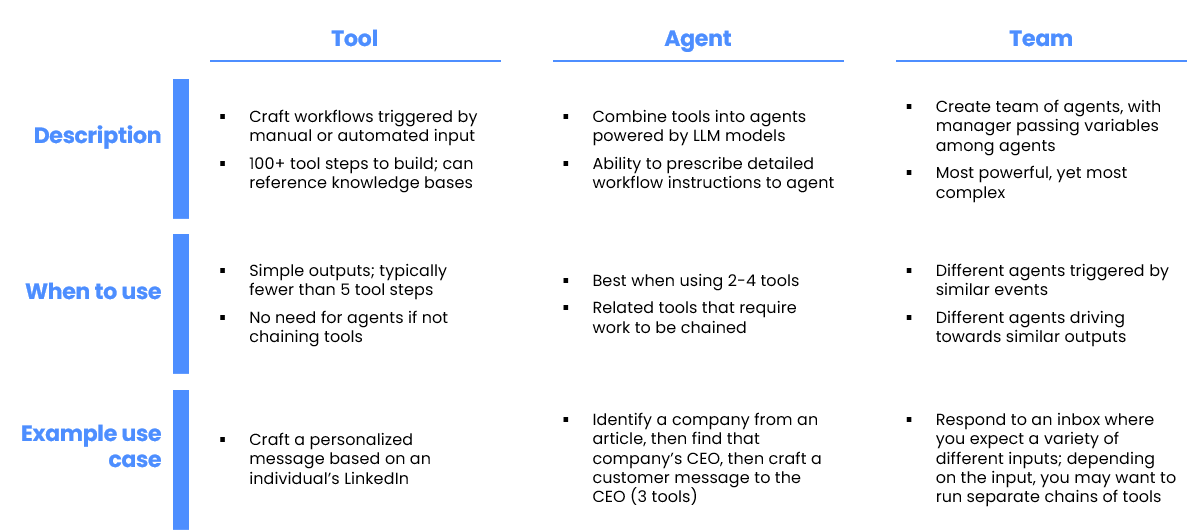
The key to maximizing accuracy when using LLMs comes from splitting large workflows / calculations into smaller stepping stones. For example, rather than ask a single tool to identify a pain point for a company based on its industry, we use two tools to identify the company’s industry and then select the matching pain point. Enabling an intelligent agent with multiple tools is more efficient and accurate than using one tool to accomplish multiple outcomes. As complexity rises, however, a single agent is no longer sufficient. Asking an agent to pick from 10+ tools makes the LLM hallucinate worse than having 9 cups of green tea.
Enter Relevance AI teams. With Relevance AI’s team structure, we create specialized bots with singular focus areas, managed by other AI-enabled agents. Furthermore, this type of hierarchy is scalable → you can have multiple managers reporting to another manager! Having left the corporate world years ago, we felt some nostalgia adding middle managers with simple functions into our workflow…
So how did we think of tasks to automate with the new teams function? Joey (our Head of Growth) and I started by asking ourselves two questions: what would we do if we had more hours in the day, and what would we want a theoretical contractor to work on that requires minimal oversight? We looked to the world’s best delegator for inspiration (the United States President), and stumbled upon a workflow titled “The Presidential Briefing.”

Verisoul’s Presidential Briefing
The Presidential Briefing is triggered automatically by our local Austin tech newsletter, the Austin Inno (s/o Brent for great daily content), through Zapier. From there, the team manager - Sequence Kick-off SK - parses the newsletter and engages the two agents that report to her team: Austin Inno Ivan and Morning News Mo. Austin Inno Ivan has a couple tools at his disposal, and he creates a table with all the companies listed in the newsletter, relevant blurbs about each company, and the CEO LinkedIns for each company. Joey and I can then shoot quick LinkedIn messages or run automated campaigns to these CEOs with hyper personalized messages tied to recent events (e.g., “Saw you just won the SXSW ‘Top EDU Start-up’ award!”).
Morning News Mo takes the date from Sequence Kick-off SK and searches Google for all relevant news articles from today by using filtered searching on relevant keywords (e.g., Fake Accounts) in Google. After scraping 30 articles, Mo uses the Verisoul knowledge base to identify the 5 most relevant articles for our team, and shoots us a slack message beginning with “Mr. President.” In addition to the incredible dopamine hit from being called “Mr. President,” we now have the most relevant news to start our day.
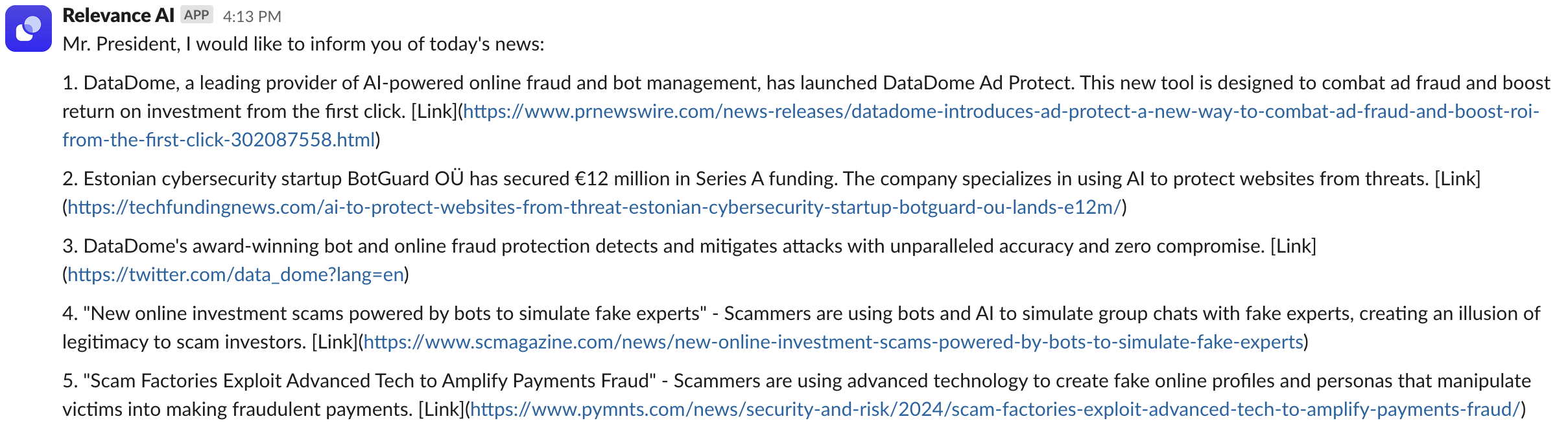
But the power of Relevance AI doesn’t stop there. If we like an article and want to leave a comment on it (without spending too much time of course), we’ll shoot a slack message to News Commander Ned, who passes the article onto his team: LinkedIn Lyndsey and Commenter Karen. Karen gives us a comment to post to the article and Lyndsey gives us the LinkedIns of our ICPs for any relevant companies referenced in the article. This entire exchange occurs in Slack.
Results and takeaways
In <5 minutes of review, we’re informed on the day’s most relevant news, have 5-10 new leads with custom outreach messages, and have a number of comments we can post to relevant articles. We’re better prepared for meetings, have a better sense of our solution’s PMF, and can run yet another automated campaign via contractors.
And we don’t have to worry about our “team” of AI Agents job hopping or applying for MBA programs (though I’d of course write a positive recommendation if Mo thought it was a good fit for his career).
Reflecting back on our first foray into using teams, we’ve got a number of tips:
- Break every task down to its simplest function. Maybe it’s splitting one agent into a team of two, or one tool into a chain of tools. This will make your life easier when your model starts to hallucinate
- The best use cases typically involve some sort of segmentation, e.g., parsing an initial input and running separate agents with different focus areas
- The more automated the workflow, the higher leverage the solution; to ensure automation, Relevance has a number of pre-built integrations, and we also set-up Zapier workflows in minutes
- When using teams, opt for the workflow functionality + variable passing as much as possible; the less specific you are, the more likely you are to get unintended results
- Have fun with the naming conventions… it’s hard to get mad at a bot named Morning News Mo, even when he’s sending you irrelevant news articles about Baby Bottle Pops
Given the perpetual, autonomous functionality, there’s not a tool we’d recommend more for high-leverage return on time than Relevance AI teams. Don’t hesitate to shoot us a note if you have questions on our Relevance AI workflow or want to see it in more detail!